Kevin Lorengel writes:

Denoising was made a lot easier in blender 2.81 when the Intel Denoiser was introduced, but what if i tell you, there is another, even better denoising method?
Super Denoising is indispensable in high-precision night shots taken with DSLr and low-light (indoors, night, no-flash, astro) and high-speed (sport, action, children) photography captured by smartphones, which offer you no control over the ISO. Super Denoising proprietary technology will ensure the best denoising results. You can choose from three zoom levels or have Super Denoising fit the photo into the app window, but it is not possible to resize the image manually. Handy utility that can reduce image noise, but would benefit from some improvements. All in all, this is not a complex application by any means, but it does the job it was designed for just fine.
The SuperImageDenoiser, or short, SID!
For a long time it was annoying and difficult to use, but now it is a super easy to use add-on that allows you to denoise your images super cleanly with minimal loss of detail!
If you want to use external programs such as AfterEffects, it can also export Multilayer-EXR files, which others can't!
It is super easy to use! You just need to press one button and its all set up for you!
Give it a try, here you can download it!
Below is a little tutorial on how to use it.
Updates are frequent! And If you suggest features, I'll see if I can add them! I am currently working on adding support for LuxCore and Radeon Pro Render, so you can enjoy detailed denoising in other render engines too!

Multilevel Encoder-Decoder Architectures for Image Restoration
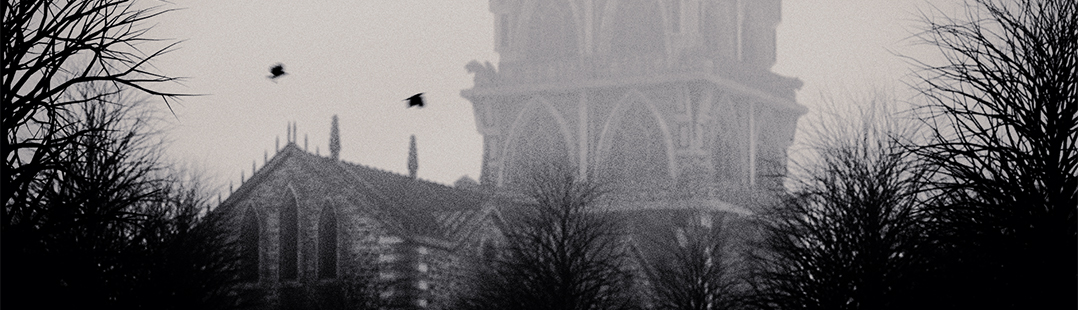
Many real-world solutions for image restoration are learning-free and based on handcrafted image priors such as self-similarity. Recently, deep-learning methods that use training data have achieved state-of-the-art results in various image restoration tasks (e.g., super-resolution and inpainting). Ulyanov et al. bridge the gap between these two families of methods (CVPR 18). They have shown that learning-free methods perform close to the state-of-the-art learning-based methods (approximately 1 PSNR). Their approach benefits from the encoder-decoder network.
In this paper, we propose a framework based on the multi-level extensions of the encoder-decoder network, to investigate interesting aspects of the relationship between image restoration and network construction independent of learning. Our framework allows various network structures by modifying the following network components: skip links, cascading of the network input into intermediate layers, a composition of the encoder-decoder subnetworks, and network depth. These handcrafted network structures illustrate how the construction of untrained networks influence the following image restoration tasks: denoising, super-resolution, and inpainting. We also demonstrate image reconstruction using flash and no-flash image pairs. We provide performance comparisons with the state-of-the-art methods for all the restoration tasks above.

Super Denoising
Super-resolution
Given a low-resolution (LR) image , and a scaling factor t, super-resolution aims to enhance image quality and generate a high-resolution (HR) image. The Tensorboard files for the results will be provided soon.
Denoising
Given a noisy image, denoising aims to reduce the noise and recover the clean image. The Tensorboard files for the results will be provided soon.
Super Denoising Software
Inpainting
Super Denoising
Inpainting involves computing missing pixel values in the corrupted image in using the correspondence of a binary mask. The Tensorboard files for the results will be provided soon.
Super Denoising Software
Flash/no-Flash
Given flash/no-flash image pair, the objective is to obtain a high-quality image which incorporates details of the scene from the flash image and ambient illumination from the no-flash image. The Tensorboard files for the results will be provided soon.
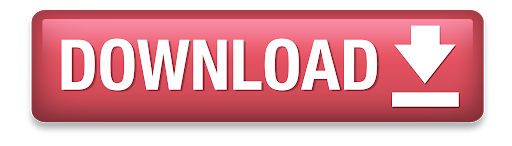